Harnessing the Power of Machine Learning Annotation Tools for Your Business
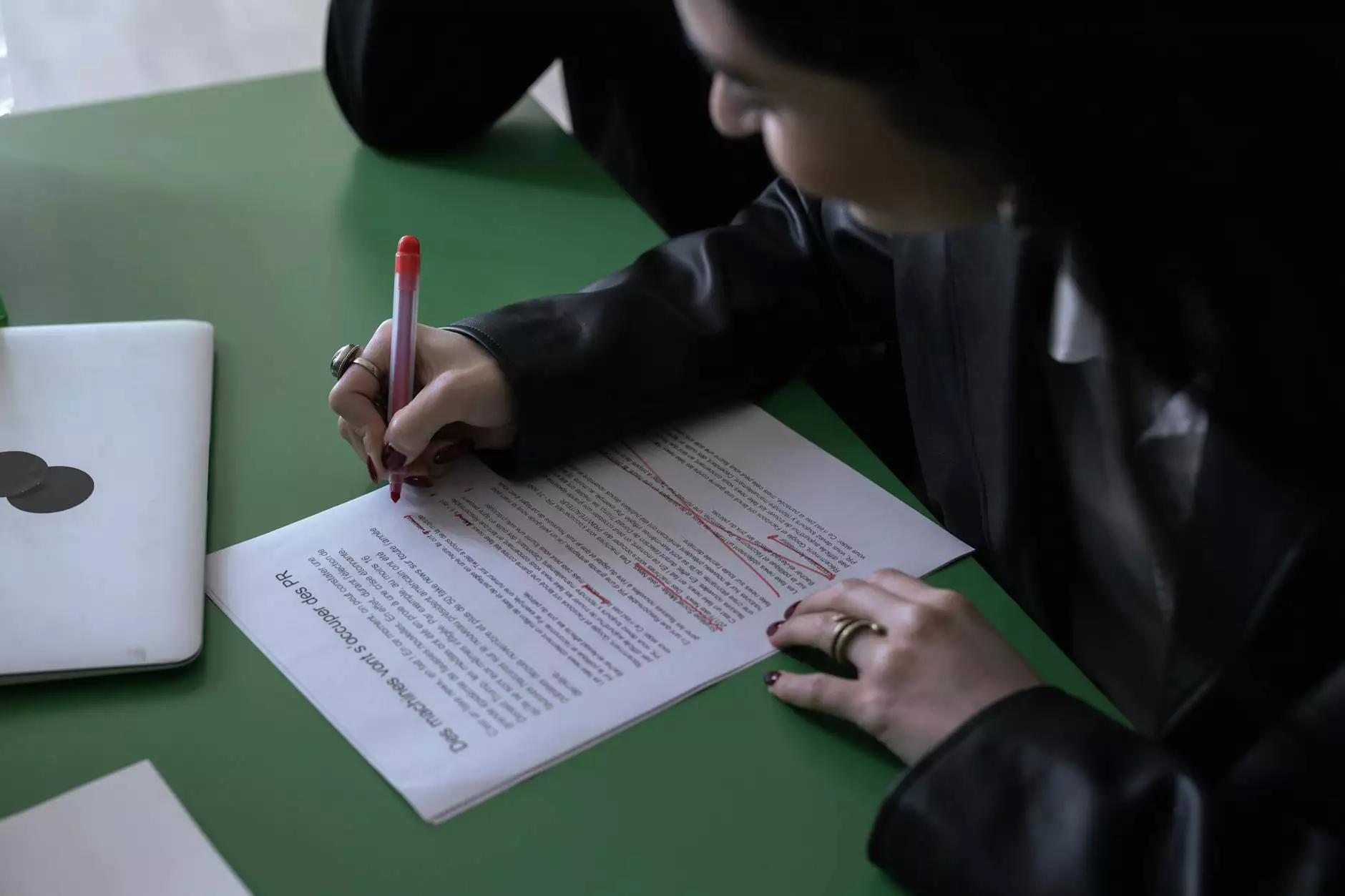
In the dynamic landscape of modern business, leveraging technology to optimize processes is not just an option—it's a necessity. Among the myriad of technologies available, machine learning annotation tools stand out for their ability to enhance data-driven decision-making.
Understanding Machine Learning Annotation Tools
Machine learning annotation tools are software applications designed to facilitate the labeling and categorization of datasets. This process is crucial, as annotated data is the backbone of effective machine learning models. These tools enable businesses to:
- Improve Data Quality: Ensuring high-quality data inputs leads to better machine learning outcomes.
- Enhance Model Performance: Correctly annotated data trains models more efficiently, leading to superior predictive capabilities.
- Streamline Workflows: Automating the annotation process can save time and resources, increasing overall productivity.
Why Machine Learning Annotation Tools Matter in Home Services and locksmiths
The industries of home services, particularly keys and locksmiths, are evolving rapidly due to advances in technology. Here’s how machine learning annotation tools contribute:
Improving Customer Service
In a service-based industry, providing top-notch customer service is paramount. Machine learning annotation tools can analyze customer interactions and feedback to identify common queries and issues. By understanding trends and patterns, businesses can:
- Anticipate Customer Needs: Predictive analytics help companies address customer issues before they escalate.
- Train Support Teams: Insights derived from annotated data lead to better training resources for staff.
- Personalize Services: Tailoring services based on customer preferences results in higher satisfaction and retention.
Optimizing Resource Allocation
Through effective data analysis enabled by machine learning tools, businesses can optimize their resource allocation:
- Data-Driven Decisions: Utilize historical data to make informed decisions about staffing and inventory.
- Efficiency Analysis: Evaluate which services are most in-demand and focus resources accordingly.
- Cost Reduction: Identify areas of wastage and streamline operations to maximize profitability.
Types of Machine Learning Annotation Tools
There are various machine learning annotation tools available in the market, each designed to cater to different needs:
Image Annotation Tools
These tools are critical for businesses that rely on visual data. They allow for the annotation of images, crucial for training computer vision models.
Text Annotation Tools
For businesses that deal with substantial text data, text annotation tools categorize and tag text, making it easier to train natural language processing models.
Audio Annotation Tools
In industries that utilize voice commands or audio data, these tools label audio clips for training voice recognition systems.
Video Annotation Tools
For businesses that require analysis of video footage, these tools help in annotating specific actions or objects in videos, enhancing object detection models.
Best Practices for Using Machine Learning Annotation Tools
To fully harness the potential of machine learning annotation tools, businesses should adhere to best practices:
1. Define Clear Objectives
Identify the specific problems you want to solve using machine learning. Clearly defined objectives help in selecting the right annotation tool and dataset.
2. Ensure Data Diversity
Diverse data helps in training robust machine learning models. Ensure your dataset covers various scenarios applicable to your services.
3. Collaborate with Experts
Involving data scientists and ML experts can enhance the annotation process, ensuring that the insights derive maximum value.
4. Regularly Update Your Data
Regular updates to your dataset keep your models relevant and effective. Continuous feedback from current operations should inform your data refresh strategies.
Future Trends in Machine Learning Annotation Tools
The future of machine learning annotation tools is bright, with emerging trends shaping how businesses operate:
Increased Automation
Automation in data annotation is set to rise, reducing manual labor while increasing accuracy.
Integration with AI Technologies
Annotation tools will increasingly integrate with AI technologies for real-time data processing and insights.
Enhanced Collaboration Features
As teams become more distributed, collaborative features within these tools will facilitate seamless interaction between annotators, managers, and stakeholders.
Conclusion: Embrace the Future with Machine Learning Annotation Tools
Businesses, especially in the home services and locksmiths sectors, have a valuable opportunity to revolutionize their operations through machine learning annotation tools. By focusing on meticulous data annotation and strategic implementation, businesses can enhance customer experiences, optimize resources, and stay ahead in a competitive market.
Investing in machine learning annotation tools is not just about keeping up with technology; it's about redefining business strategies for sustained growth and success. By adopting these tools, you position your business to thrive in an increasingly data-driven world.