Unlocking the Future of **Data Labeling Machine Learning**
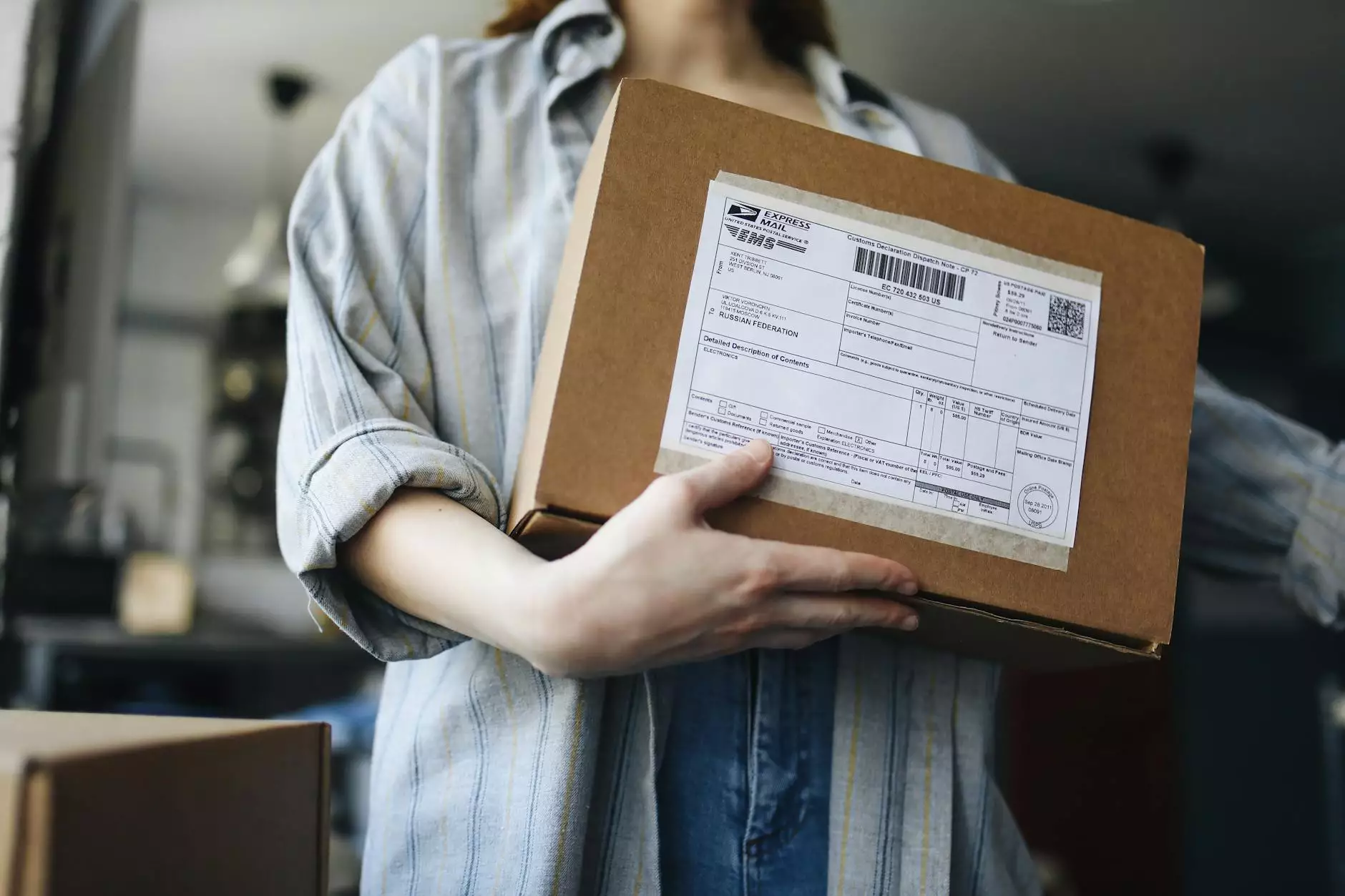
The advent of data labeling has revolutionized the field of machine learning (ML), enabling businesses to harness the power of data effectively. In today’s technology-driven world, the role of data annotation tools and platforms has become increasingly crucial, especially for organizations aiming to develop sophisticated AI systems. This article will explore the essential aspects of data labeling in machine learning, detailing its benefits, techniques, platforms, and the future of this burgeoning field.
What is Data Labeling?
Data labeling refers to the process of tagging and annotating data to facilitate machine learning models’ understanding of information. In simpler terms, it involves identifying relevant data points and categorizing them appropriately. This process is fundamental as ML algorithms rely on labeled data to learn patterns, make predictions, and improve their accuracy over time.
Importance of Data Labeling in Machine Learning
Data labeling is vital for any successful machine learning project. Here are several reasons highlighting its importance:
- Quality of Data: Labeled data improves the quality of machine learning models, ensuring they are trained on high-quality, accurate datasets.
- Model Performance: The better the data is labeled, the higher the model’s ability to generalize from training data to unseen scenarios.
- Informed Decisions: Leveraging accurately labeled data helps businesses make informed decisions based on reliable MT outcomes.
- Scalability: As businesses grow, the volume of data increases, necessitating efficient labeling processes for scalability.
Types of Data Labeling Techniques
Different techniques are employed for data labeling, each suited for various types of data and use cases. Here is an overview of some commonly used techniques:
1. Image Annotation
Image annotation involves tagging specific elements within images. This technique is essential for computer vision tasks such as object detection, image segmentation, and facial recognition. Annotation methods can include bounding boxes, polygons, landmarking, and more.
2. Text Annotation
This involves labeling text data to facilitate natural language processing (NLP) tasks. Common forms of text annotation include sentiment analysis, entity recognition, and parts of speech tagging.
3. Audio Annotation
In this method, audio files are transcribed and labeled to train speech recognition systems or sentiment analysis models. This process can include time-stamping specific phrases or categorizing sounds.
4. Video Annotation
Video annotation consists of labeling frames in a video stream for applications such as activity recognition and automated surveillance. Techniques include frame-by-frame labeling and trajectory tracking.
Key Benefits of Using Data Annotation Tools
Harnessing the right data annotation platform can significantly enhance the data labeling process. Let’s explore a few benefits:
- Increased Efficiency: Automated tools can drastically speed up the labeling process, allowing businesses to handle large datasets without compromising quality.
- Cost-Effectiveness: By optimizing the labeling process, organizations can reduce the costs associated with data preparation.
- Improved Accuracy: Many annotation tools use advanced algorithms to ensure high accuracy in labeling, thus reducing human error.
- Scalability: A reliable data annotation tool can easily scale with increasing data volumes, accommodating the growth of your business.
Choosing the Right Data Annotation Platform
When selecting a data annotation platform, businesses should consider several factors:
1. Data Type Compatibility
Ensure the platform supports the type of data you plan to annotate, such as images, text, audio, or video. Accurate annotation depends on compatibility.
2. User Experience
Evaluate the usability of the platform. A user-friendly interface ensures that your team can utilize the tool effectively without extensive training.
3. Quality Control Features
Look for platforms that offer built-in quality control measures, allowing for the verification of labeled data and minimizing errors.
4. Integration Capabilities
The ability to integrate with existing systems and workflows can enhance operational efficiency. Consider platforms that seamlessly connect with your current tech stack.
Challenges in Data Labeling
Despite its significance, data labeling comes with challenges, including:
- Subjectivity: Annotation can sometimes be subjective, leading to inconsistencies in labeled data.
- Scalability Issues: As data grows, maintaining quality and speed in labeling can become challenging.
- Resource-Intensive: The process often requires substantial human resources, which may not always be readily available.
Future Trends in Data Labeling for Machine Learning
The future of data labeling in machine learning is promising, with several trends shaping its evolution:
1. Artificial Intelligence and Automation
AI is poised to play a substantial role in data labeling, with automated tools emerging to reduce the burden of manual labeling. Automated systems can significantly speed up the process while maintaining high accuracy.
2. Crowdsourcing
Crowdsourcing data annotation is gaining traction, enabling businesses to tap into the collective expertise of a diverse workforce. This approach can enhance scalability and reduce costs.
3. Contextual Labeling
Improved contextual algorithms are being developed to provide more meaningful labeling based on content understanding, thus enhancing data utility.
Conclusion
As businesses continue to embrace the power of machine learning, the demand for effective data labeling processes and tools will only grow. Embracing innovative data annotation platforms not only accelerates model training but also enhances the accuracy of insights drawn from data. Companies like KeyLabs.ai are at the forefront of this transition, providing cutting-edge solutions to ensure your data is optimized for success.
In conclusion, investing in quality data labeling is not just an operational necessity but a strategic advantage in the competitive business landscape of machine learning. By understanding and leveraging the transformative power of data labeling, organizations can unlock profound insights and drive extraordinary results.
data labeling machine learning